Quandela in collaboration with Quantinuum have just announced a breakthrough in photonic quantum computing, potentially accelerating the development of practical quantum algorithms. This innovation addresses a crucial challenge in optimizing quantum algorithms on photonic platforms, making them more efficient and reliable.
Why This Matters
Imagine trying to navigate a complex landscape blindfolded – that’s similar to what quantum computers face when trying to optimize a variational algorithm without proper gradient information. Until now, photonic quantum computers had to rely on less efficient methods because they couldn’t calculate gradients as easily as their qubit-based cousins. This discovery extends a reliable approach for calculating gradients on quantum computers for photonic devices.
The Challenge
Gradients are usually calculated via the finite difference scheme, where a precise computation requires small changes of circuit parameters. However such method becomes unsuitable for current quantum hardwares, due to the inherent noise in NISQ devices. To address this, a method called “Parameter-Shift Rules” (PSR) has been developed to compute the gradient of a quantum circuit without relying on small variations on the circuit. As such, a gradient of a circuit can be calculated using the same circuit evaluated on different shifted values of the parameters, hence the name of the method. Until now, these recipes have been only studied in the context of qubit-based quantum computers.
On the other hand, Photonic quantum computers use light particles (photons) to process information, offering several advantages like:
- Operating at room temperature
- Natural connectivity for quantum networks
- Lower maintenance requirements compared to other quantum technologies
The manipulation of the information carried by photons is very different from the information processing on qubit-based quantum computers. Therefore, the computation of gradients for qubit-based Variational Quantum Algorithms (VQAs), which are considered some of the most promising applications for near-term quantum computers, was not directly suited to photonic-native VQAs.
The Innovation: A Photonic Parameter-shift Rule
We have developed what they call a photonic Parameter-Shift Rule. This mathematical technique allows photonic quantum computers to calculate exact gradients for optimizing algorithms. Here’s why it’s special:
- Exact Calculation: Unlike alternative methods that gave approximate results, this new approach provides exact gradient computations.
- Efficiency: The method scales linearly with the number of input photons, making it practical for real-world applications.
- Practical Implementation: It uses the same circuit with different parameter settings, making it hardware-efficient.
- Noise Resistance: The approach shows remarkable robustness against common sources of noise in photonic systems, including:
- Finite sampling errors
- Photon distinguishability issues
Results and Real-World Applications
The team demonstrated their method’s effectiveness in two key areas:
- Quantum Chemistry: Using their technique for a Variational Quantum Eigensolver (VQE) to calculate molecular properties, showing better convergence than traditional methods (see Figure 1).
- Machine Learning: Implementing a Quantum Circuit Born Machine (QCBM) for generative modeling tasks, demonstrating superior optimization performance (see Figure 2).
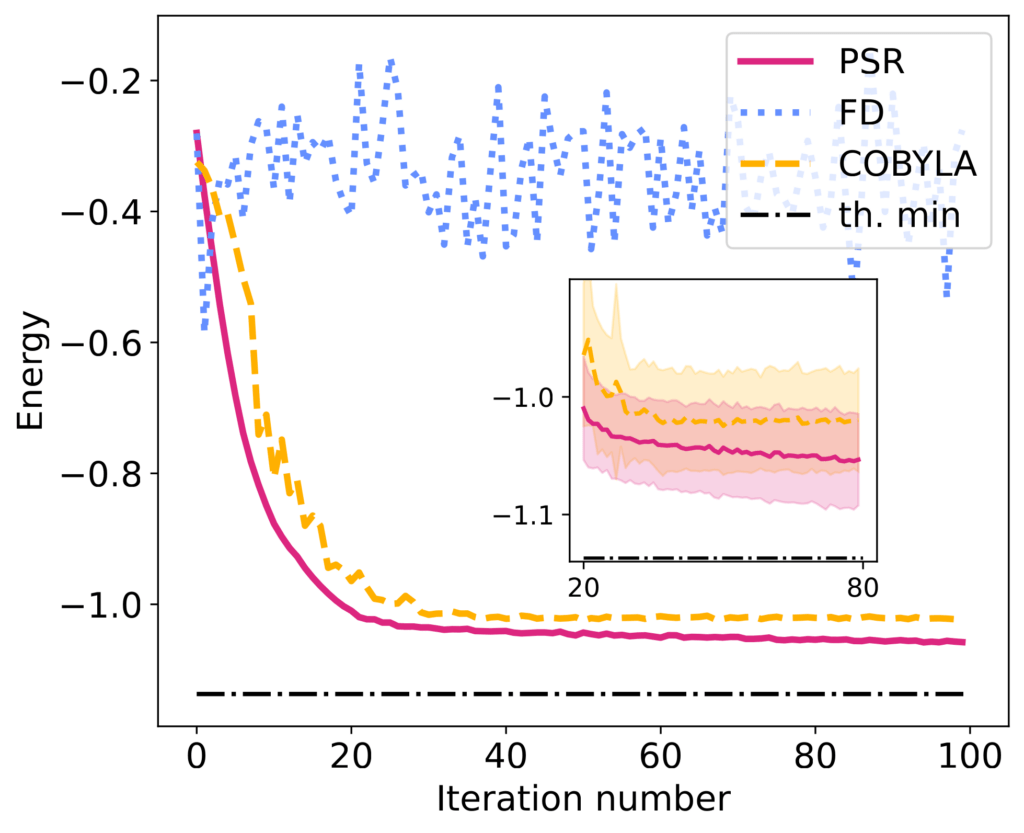
Figure 1: VQA for ground state energy calculation of the $H_2$ molecule. A realistic noisy scenario has been crafted using finite sampling and presence of photon distinguishability.
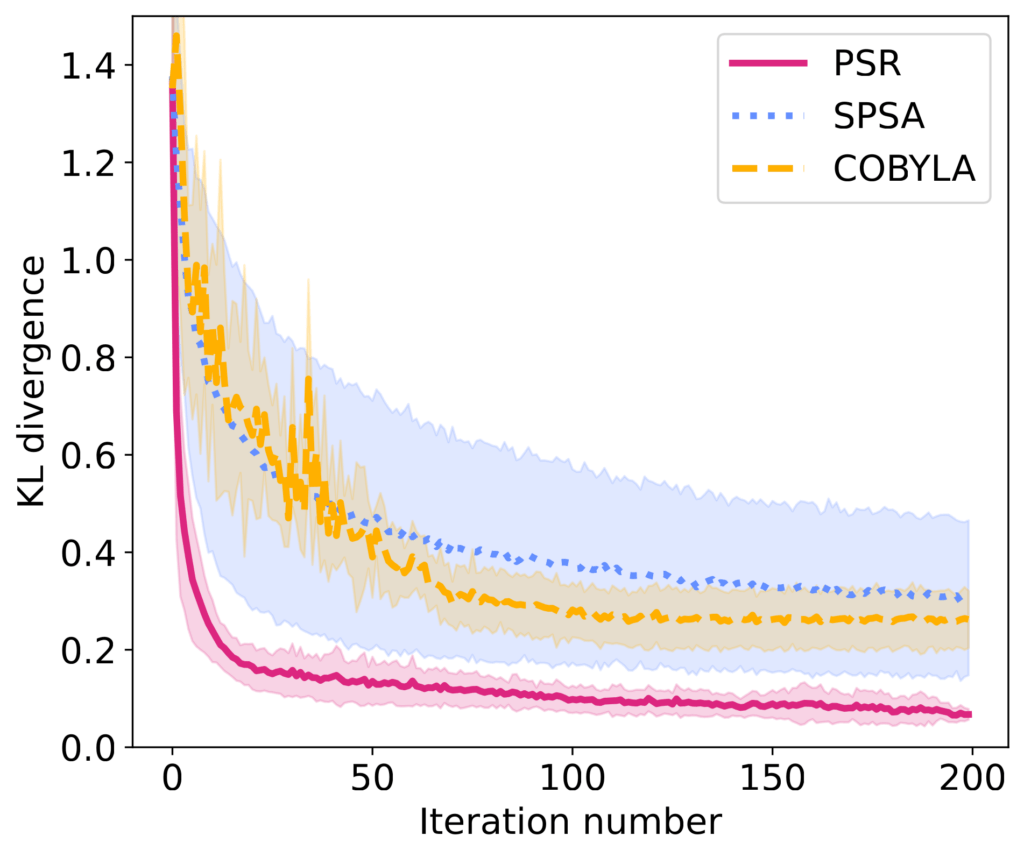
Figure 2: VQA for optimising a QCBM instance. The same noisy settings as in Figure 1 was used.
This development bridges a crucial gap between photonic and gate-based quantum computing platforms. Previous optimization methods for photonic quantum computers were either:
- Too sensitive to noise (like finite differences)
- Slow to converge
- Prone to getting stuck in local optima
The new photonic PSR combines the best of both worlds: the precision of gradient-based methods with the robustness needed for practical quantum computing.
What comes next ?
This breakthrough opens new possibilities for photonic quantum computing, particularly in:
- Near-term quantum applications
- Variational quantum algorithms
- Quantum machine learning
- Quantum chemistry simulations
As quantum computers continue to evolve, this development could prove crucial in making photonic platforms more practical and efficient for real-world applications.
Impact on the Field
This research represents a significant step towards making photonic quantum computing more practical and efficient. By solving the gradient computation problem, it removes a major roadblock in the development of useful quantum algorithms on photonic platforms.
The combination of photonic quantum computing’s inherent advantages with this new capability for efficient optimization could accelerate the development of practical quantum applications across various fields, from chemistry to machine learning.